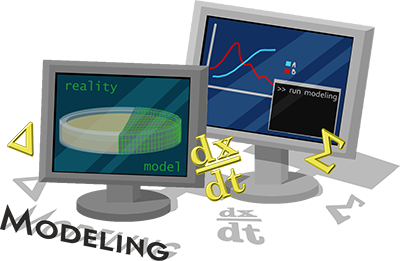
Guide
Our model can be divided into three parts which are closely linked with our project and wet-lab experiments as follows:
1. Reduce tooth decay by controlling population size of dominant dental plague, S. mutans.
>> Growth & pH
In this model, we want to know the relation between dental plague and tooth decay. Since tooth decay is the consequence of acid producing from bacteria metabolism, it’s important to know how pH related to bacteria growth.
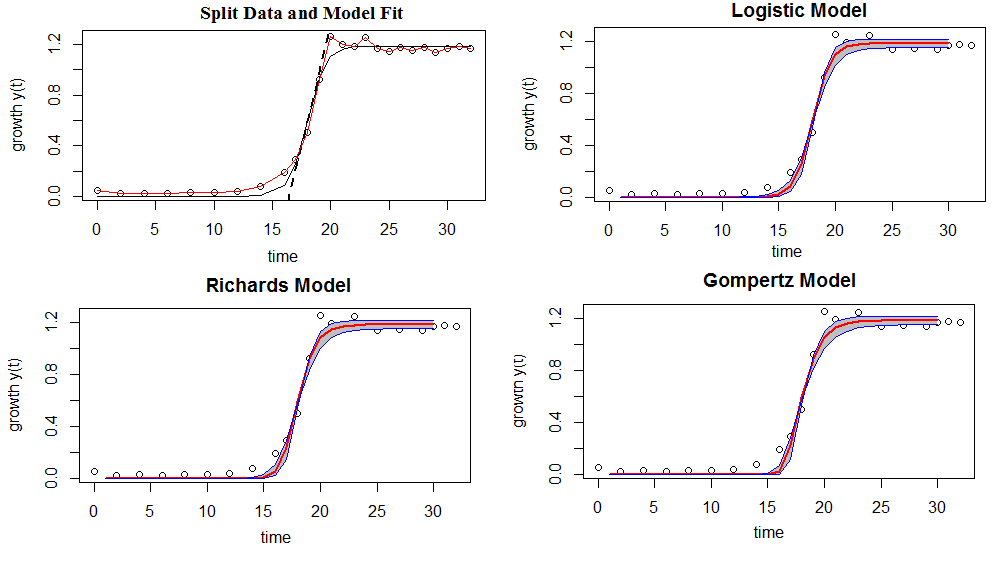
>> Competition
There are several species of dental plague. Though Streptococcus mutans is the dominant one, changing one species’ quorum may impact the whole balance of the ecology. The result shows different balance of some oral microbial ecology by changing the population size of S. mutnas, and it concludes how to control S. mutans may lead to the lower acid producing.
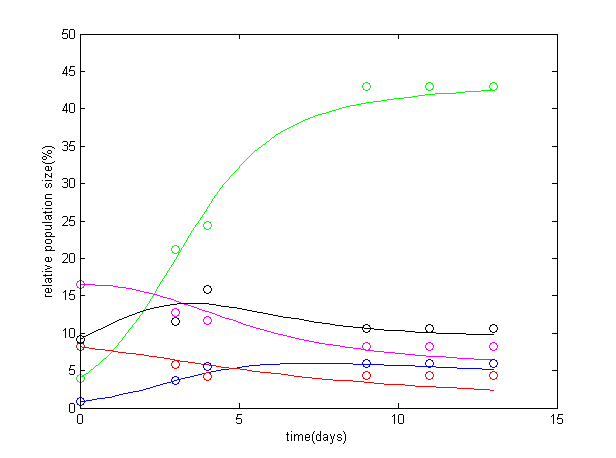
>> Threshold
This model describes a key circuit of the system. It is a threshold-control circuit which has killing protein after the sensing promoter and terminators. It shows how different terminators are combined can regulate the protein expression.
2. Predict how our product of project might work in a real circumstance.
>> Stephen curve
In dentistry, the way to measure tooth decay is highly related to pH level change in oral. The result compares the original balanced ecology and altered one, to see if controlling S. mutans quorum can reduce the risk of tooth decay in a dental point of view.
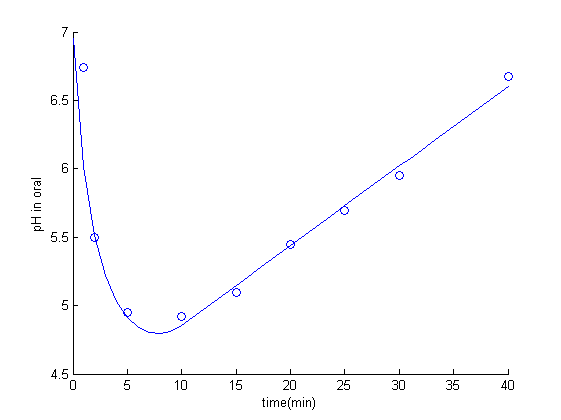
>> Effectiveness
Cooperated with our human practice questionnaire and statistics of decay population in each age group, the result shows if our project is helpful in lowering the decay rate.
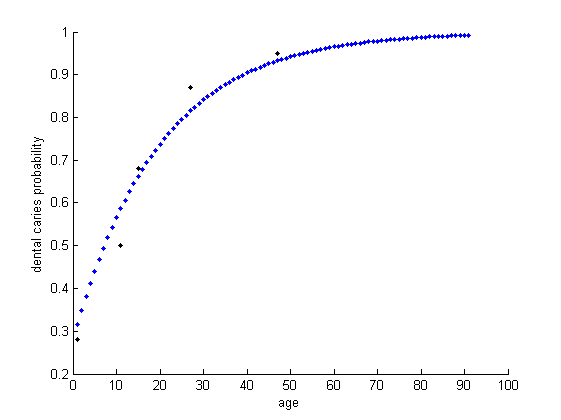
3. Simulate some of our new parts with different ways.
>> New part 1
For Target target, because the functional testing requires a combination of many experiment conditions, which is difficult for us to operate, we model the protein expression amount by using the parameters we found in the literature since they were commonly used.

>> New part 2
For Attachment part, the circuit we have finished functional test, we do data fitting and found estimated value of the parameters. The promoter strength, translation rate, or degradation rate we derived may be useful for future teams when modeling INPNC circuit.
What’s new or special in our model
NYMU IGEM modeling team this year has made a lot of effort to do modeling based on real experimental data, from our wet lab team or from literature. To our knowledge, numerous iGEM teams do modeling without experimental data, either by finding parameters in literature or do parameters sweeping. We have tried modeling in this way but found that parameters differ in different paper, as the experiment conditions are not the same. As a result, we think this may lead to some error and if we obtain parameters from separate articles and put it together for modeling. However, since lots of us, the iGEMers, are constructing new combinations of gene or doing something that is totally new, it is nearly impossible for us to find a set of suitable parameters in one study. Thus, we suggest all the future iGEM teams be careful about the parameters they use, and we think that fitting parameters and building models based on experimental data is really a good way to avoid establishing unrealistic models.
When fitting data, we used the so called Genetic Algorithm, which is a useful solver for optimization and search problems, but is merely used by iGEMers when doing data fitting. Genetic Algorithm is a method for solving constrained or unconstrained optimization problems based on natural selection and biological evolution. It iterates for modifying a population of solutions. Similar to biological reproduction, the genetic algorithm selects individuals at random from the current population to be parents and uses them to produce the children for the next generation. The children are selected based on fitness function, the one that represents the optimization problem. Thus, the population would evolve toward an optimal solution like population genetics in living creatures.
For data fitting and parameter estimating in modeling, our fitness function is the sum of quadratic difference between simulated and experimental result. The optimal parameters having the least square error would be the solution of the algorithm. We use this method because its performance is much better than other algorithm and we then recommend this method to future iGEM team when doing data fitting.